De novo design of high-affinity binders of bioactive helical peptides.
Publication Type:
Journal ArticleSource:
Nature (2023)Abstract:
<p>Many peptide hormones form an alpha-helix upon binding their receptors, and sensitive detection methods for them could contribute to better clinical management of disease. De novo protein design can now generate binders with high affinity and specificity to structured proteins. However, the design of interactions between proteins and short peptides with helical propensity is an unmet challenge. Here, we describe parametric generation and deep learning-based methods for designing proteins to address this challenge. We show that by extending RFdiffusion to enable binder design to flexible targets, and to refining input structure models by successive noising and denoising (partial diffusion), picomolar affinity binders can be generated to helical peptide targets both by refining designs generated with other methods, or completely de novo starting from random noise distributions. To our knowledge these are the highest affinity designed binding proteins against any protein or small molecule target generated directly by computation without any experimental optimisation. The RFdiffusion designs enable the enrichment and subsequent detection of parathyroid hormone and glucagon by mass spectrometry, and the construction of bioluminescence-based protein biosensors. The ability to design binders to conformationally variable targets, and to optimise by partial diffusion both natural and designed proteins, should be broadly useful.</p>
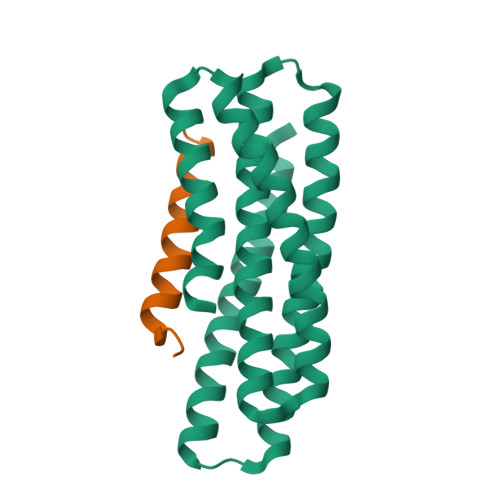